5 1 Factorial Designs with Two Treatment Factors STAT 503
Table Of Content
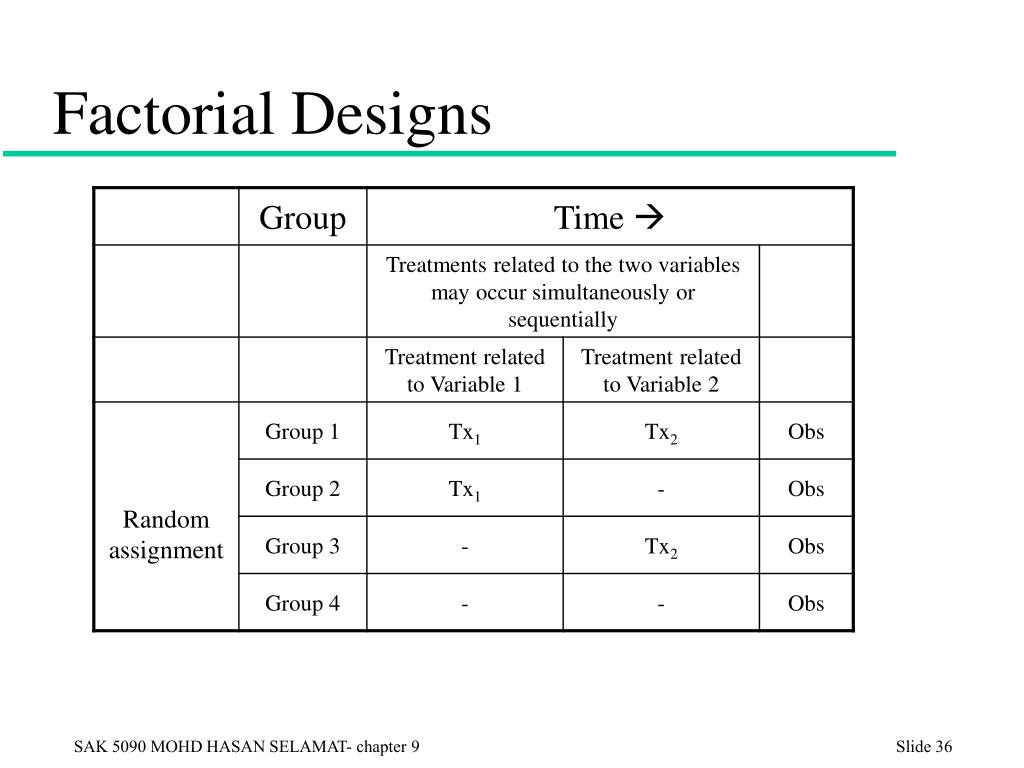
When we look at the normal probability plot below, created after removing 3-way and 4-way interactions, we can see that now BD and BC are significant. The surface plot shows us the same interaction effect in three dimensions in the twisted plane. In addition you can ask Minitab to provide you with 3-D graphical tools that will allow you to grab these boxes and twist them around so that you can look at these boxes in space from different perspectives. These procedures are all 'illustrated in the "Inspect" Flash movie at the beginning of this section. In visually checking the residuals we can see that we have nothing to complain about. There does not seem to be any great deviation in the normal probability plot of the residuals.
Assigning Participants to Conditions
Complex correlational research, however, can often be used to rule out other plausible interpretations. Research findings are often presented to readers using graphs or tables. For example, the very same pattern of data can be displayed in a bar graph, line graph, or table of means. These different formats can make the data look different, even though the pattern in the data is the same. An important skill to develop is the ability to identify the patterns in the data, regardless of the format they are presented in. Some examples of bar and line graphs are presented in the margin, and two example tables are presented below.
Basic Elements of RCT and Factorial Designs
Dr. Loh conducts research and consults for the pharmaceutical industry on statistical methodology, but the activities are unrelated to smoking or tobacco dependence treatment. Everyone gets the same pictures and does the same thing, so we're not actually testing anything. Since this is a first order, linear model, the coefficients can be combined with the operating parameters to determine equations. The next step is selecting which terms will be analyzed for the responses. To do this, click on "Terms..." and the following menu will appear.
Factorial Designs
When you have a non-parametric situation you may have a different shaped distribution in different parts of the experiment. You have to be careful about using the mean in one case, and the media in another ... For those of you who have studied heteroscedastic variance patterns in regression models you should be thinking about possible transformations. There is a gap in the histogram of other residuals but it doesn't seem to be a big problem. Let's use the dataset (Ex6-2.csv) and work at finding a model for this data with Minitab...
2. Multiple Independent Variables¶
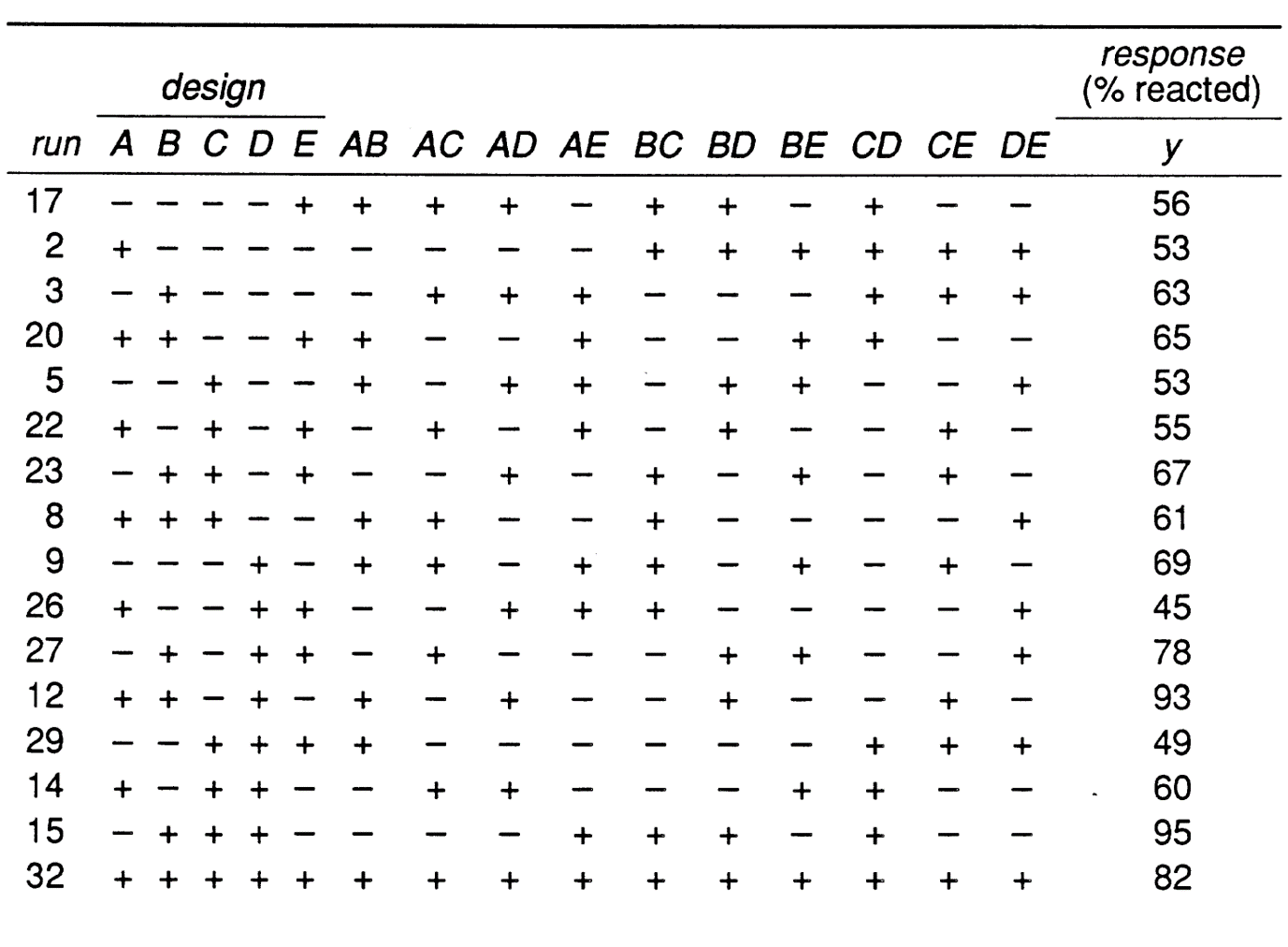
In addition to the above effects plots, Minitab calculates the coefficients and constants for response equations. The response equations can be used as models for predicting responses at different operating conditions (factors). The coefficients and constants for wt% methanol in biodiesel and number of theoretical stages are shown below. The following Yates algorithm table was constructed using the data from the interaction effects section.
Researchers want to determine how the amount of sleep a person gets the night before an exam impacts performance on a math test the next day. But the experimenters also know that many people like to have a cup of coffee (or two) in the morning to help them get going. The point of this example is that although the B factor is not significant as it relates to the response, percentage of product defects - however if you are looking for a recommended setting for B you should use the low level for B. However, by choosing B at the low level you will produce a more homogeneous product, products with less variability. What is important in product manufacturing is not only reducing the number of defects but also producing products that are uniform.
We have discussed how the manipulation of multiple treatment factors might create unintended effects due to overall burden, inducement of optimism, apparent incompatibility of components or delivery routes, differential staff delivery, and so on. Investigators should consider using measures that would be sensitive to such effects. For instance, investigators might assess measures of burden (treatment fatigue) and determine if these are especially highly related to particular ICs or to an increasing number of ICs.
Multivariate experimental design provides insights for the optimisation of rechloramination conditions and water age to ... - ScienceDirect.com
Multivariate experimental design provides insights for the optimisation of rechloramination conditions and water age to ....
Posted: Fri, 11 Mar 2022 18:06:31 GMT [source]
2.5. Graphing the Results of Factorial Experiments¶
In a within-subjects factorial design, all of the independent variables are manipulated within subjects. All participants could be tested both while using a cell phone and while not using a cell phone and both during the day and during the night. This would mean that each participant would need to be tested in all four conditions. The advantages and disadvantages of these two approaches are the same as those discussed in Chapter 5.
Box Behnken design-based optimized extraction of non-dioxin-like PCBs for GC-ECD and GC-MS analyses in milk ... - ScienceDirect.com
Box Behnken design-based optimized extraction of non-dioxin-like PCBs for GC-ECD and GC-MS analyses in milk ....
Posted: Thu, 20 Aug 2020 06:24:27 GMT [source]
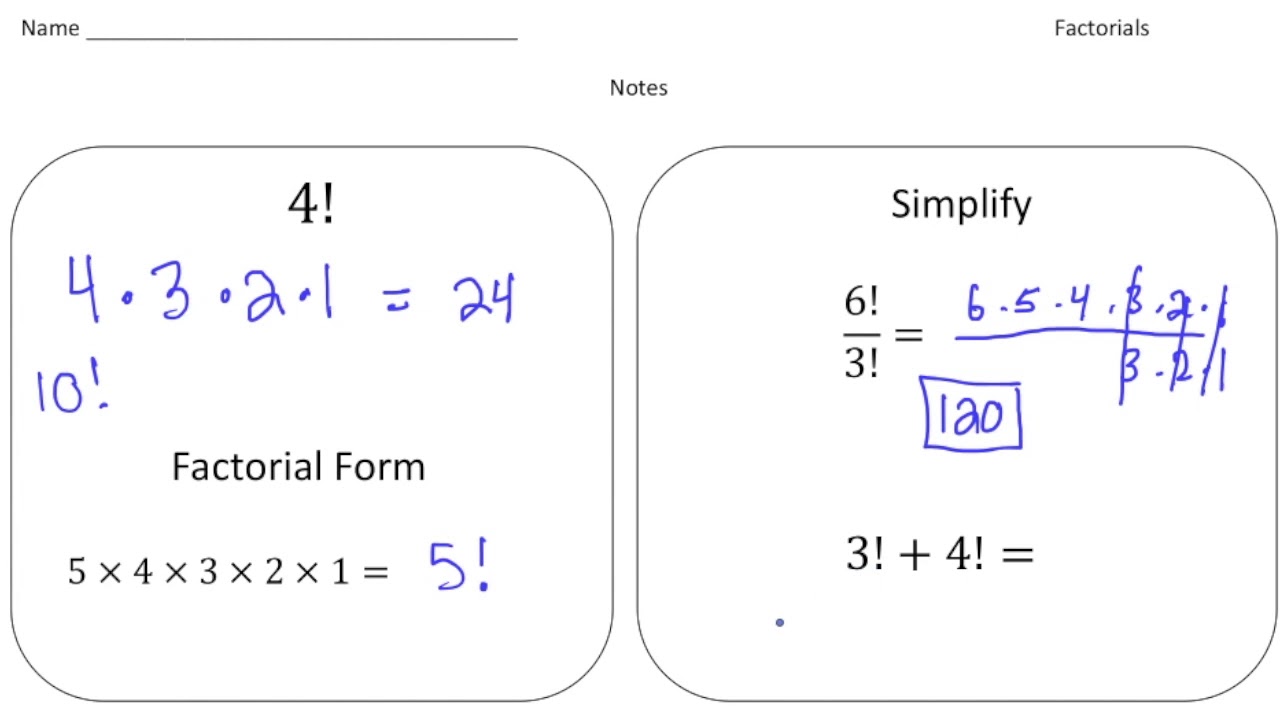
The first step in analyzing the results is entering the responses into the DOE table. In the columns to the right of the last factor, enter each response as seen in the figure below. The above table contains all the conditions required for a full factorial DOE. Minitab displays the standard order and randomized run order in columns C1 and C2, respectively. The first run (as specified by the random run order) should be performed at the low levels of A and C and the high levels of B and D. In the main "Create Factorial Design" menu, click "OK" once all specifications are complete.
Except in this case, we find the average heights in the no hat vs. hat conditions by averaging over the shoe variable. To find the main effect of the shoes manipulation we want to find the mean height in the no shoes condition, and compare it to the mean height of the shoes condition. To do this, we collapse, or average over the observations in the hat conditions. For example, looking only at the no shoes vs. shoes conditions we see the following averages for each subject. A traditional experiment would involve randomly selecting different tanks of fish and feeding them varying levels of the additive contained within the feed, for example none or 10%.
If the p-value for the interaction test is greater than 0.25 then you can drop the interaction term. Statistical theory shows that in general dropping the interaction term increases your false rejection rate for subsequent tests. Hence we usually do not drop nonsignificant terms when there are adequate sample sizes. However, if we are doing an independent experiment with the same factors we might not include interaction in the model for that experiment.
One example of a crossover interaction comes from a study by Kathy Gilliland on the effect of caffeine on the verbal test scores of introverts and extraverts [Gil80]. Introverts perform better than extraverts when they have not ingested any caffeine. But extraverts perform better than introverts when they have ingested 4 mg of caffeine per kilogram of body weight. There is an interaction effect (or just “interaction”) when the effect of one independent variable depends on the level of another. Although this might seem complicated, you already have an intuitive understanding of interactions.
These included health, knowledge of heart attack risk factors, and beliefs about their own risk of having a heart attack. They found that more optimistic participants were healthier (e.g., they exercised more and had lower blood pressure), knew about heart attack risk factors, and correctly believed their own risk to be lower than that of their peers. This kind of design has a special property that makes it a factorial design.
Counselors could either reduce the intensity of an intervention component when it is one of many that a participant receives, or they could increase the intensity of an intervention component if the participant is receiving little other treatment. In short, maintaining treatment delivery fidelity may take more care, training and supervision in a factorial experiment than in an RCT. One of the big advantages of factorial designs is that they allow researchers to look for interactions between independent variables. One common type of experiment is known as a 2×2 factorial design. In this type of study, there are two factors (or independent variables), each with two levels. These ideas can be confusing if you think that the word “independent” refers to the relationship between independent variables.
Comments
Post a Comment